Understanding the Risk Profile: The Most Important Step in Insurance Decisions
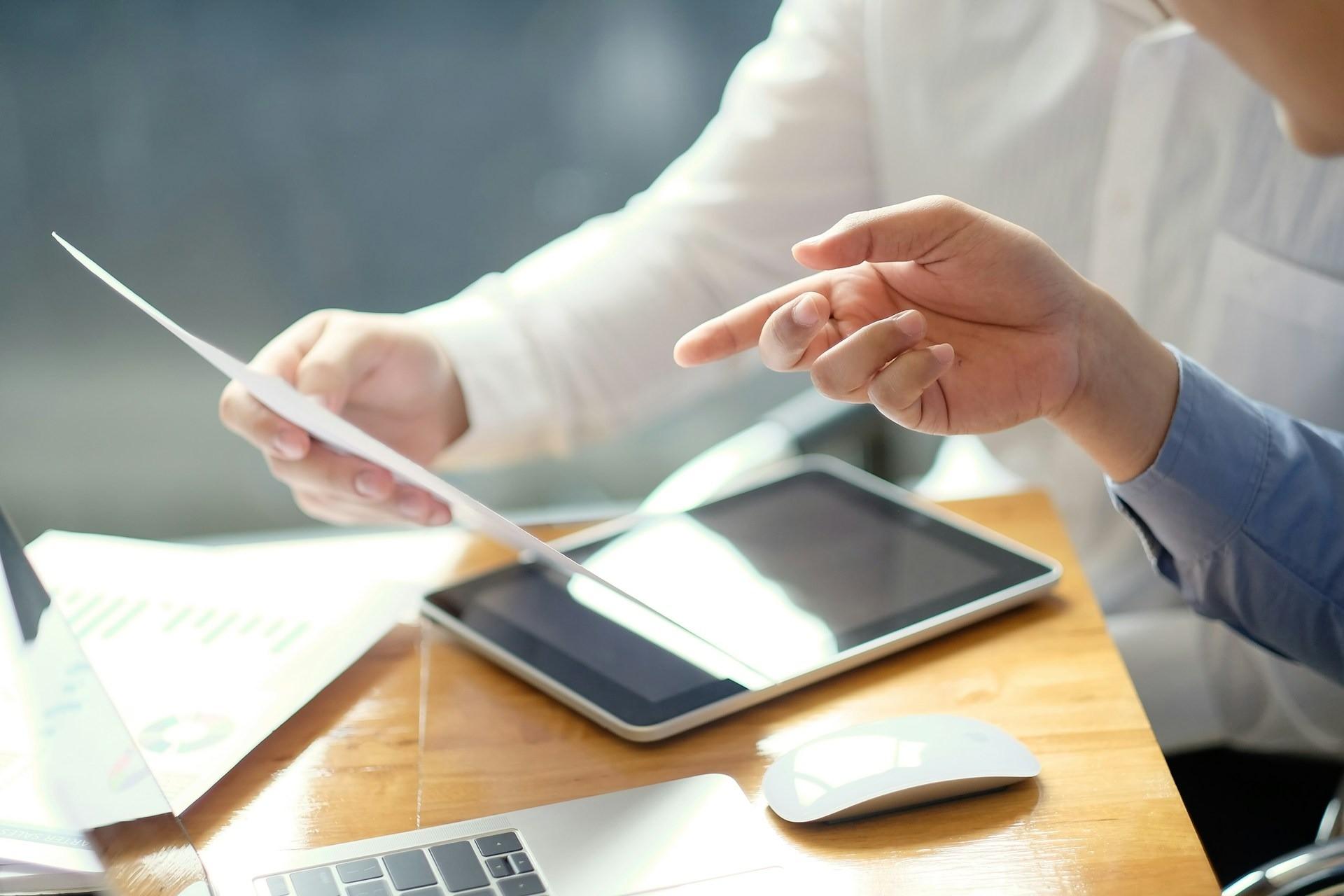
In 2021, the risk team implemented a quantitative risk-based approach to insurance renewals, which reduced the cost of insurance by 60% and improved the quality of coverage across all insurance lines. This approach allowed us to save, or rather pay a fair price, for insurance worldwide. The key to success was not to rely on market “best practices” but on testing various hypotheses in the market and relying on data.
Understanding the Risk Profile: Framing the Problem
The first and probably the most important step is understanding the underlying risk profile. This is what, in decision science, would be called framing the problem. For example, once we started investigating every insurance renewal, we discovered that:
- There was no evidence to continue insuring some risks at all, given the exposure being within shareholder risk appetite
- Limits were often completely disconnected from actual risk scenarios (usually underinsurance on property and cargo and over-insurance on liability lines)
- Low deductibles made no economic sense given the cost of transfer and the cost of retention of risk.
- Low loss ratios on some of the lines which needed to be investigated.
Compulsory vs. Voluntary Insurance
We start by separating compulsory and voluntary insurance. Since compulsory insurance in various countries is usually heavily regulated, including pricing, we try to switch to longer-term policies and don’t waste time on renewals every year.
On the voluntary insurance lines, we start with a discussion with the business and the internal customer to find out the reasons for having insurance, historical losses, perceived risk profile and other relevant information.
Sometimes we find out that insurance is a requirement from customers, business partners, contractors, banks or a regulatory requirement imposed in some countries and then we switch our quant risk analysis hat on.
We are very selective where it makes sense to quantify risks since it usually takes a lot of time, months of work in fact. Spoiler alert, it is totally worth it.
Data Collection and Market Engagement
We spend months collecting internal data and consulting with relevant risk experts before contacting insurance markets. Once we have built a decision tree or a bow-tie diagram and a simple model for the risk we reach out to external sources – underwriters, brokers, insurers, reinsurers, rating and analytical agencies, to collect market claims data and any other relevant information. We will need external data later to compare our company risk profile against the industry and to add tail events to our model.
For example, for cargo insurance, we investigated and risk assessed which cargoes were being transported (reviewed lots of MSDS): which routes, which ports, which ships, which risks are the most significant to the other market players, which risks are the most significant for us, what controls we have in place over these risks.
Then, we analyze internal and external statistics and model the distribution of expected losses for this type of risk. We could use Poisson distribution for the frequency of events and Pareto or Lognormal for the consequences.
Sometimes, it would make sense to use a splice distribution, with GPD used to model the tail of the distribution. The limit is determined from a worst-case scenario, usually losing the biggest ship with the most expensive product transportable on that ship, given the volatility of prices.
For other insurance types, we often engage external risk engineers to estimate plausible worst-case scenarios. It turned out that what is considered industry best practices was absolutely useless to us in quantifying risk profile, so we ended up writing a whole new technical specification for the risk survey from scratch.
Sometimes, after the initial risk analysis, we would come back to business with the conclusion that insurance may not be required at all, as risk is within risk appetite and request additional information if the business insists on insurance.
Recently, the customer (internal risk owner) said that he wants the same insurance limit as last year. Having collected the data, we saw that there was no evidence to suggest the same limit was necessary and that a limit four times lower would cover the risk at the given confidence level.
Conclusion
Our journey reveals that a data-driven approach, while resource-intensive, provides immense value. It allows us to challenge norms, push boundaries, and ensure fair and effective insurance pricing.
Are you ready to transform your professional future with AI-driven insights? Join us at RAW2024, the premier event for risk management professionals eager to harness the power of AI and data-informed strategies. Whether you’re looking to climb the career ladder, enhance your team’s capabilities, or pivot into a role where risk management takes center stage, RAW2024 is your catalyst for success.